Nowadays, banks and financial institutions employ a growing wider range of decision making tools. Data gathering and analysis has empowered these organizations to better identify opportunities and estimate the related risks. It is a complex landscape, influenced by many different factors and subjects: technical advancements are pushing the limits of IT transformation and automation in the sector, and banks are increasingly required to answer to shareholders and authorities.
One of the areas in which banks and institutions face increasing requirements is that of credit risk, presenting challenges and responsibilities that financial organizations are expected to match. According to a recent analysis of the credit risk landscape by the European Central Bank’s (ECB), nearly one in five of all deficiencies identified
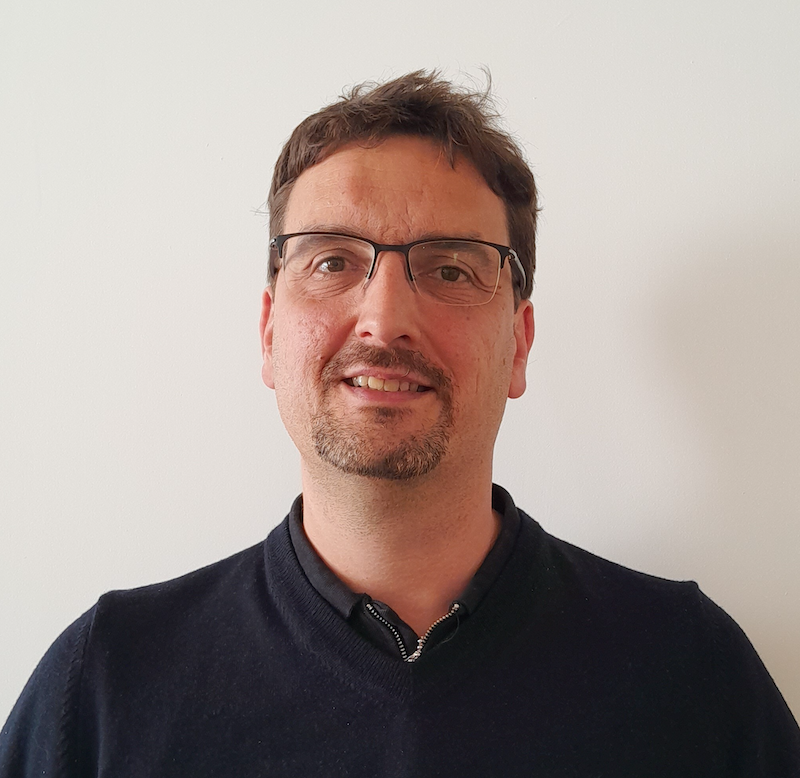
related to credit risks; 44 percent of these represented shortcomings in banks’ credit risk management frameworks, a third related to non-performing exposures (NPEs), and another 10 percent related to overall loan classification and provisioning.
The evolving value of data in credit scoring
Credit scoring is the outcome of a financial analysis to decide whether to extend a loan to an individual or a business by assessing the risks of default and determining the most appropriate loan amount and interest rate for that risk.
Credit scoring is performed on private data using machine learning algorithms. The data is typically collected by a credit bureau and the lender (bank or supplier) extending the loan or credit. Credit bureau would have general information about the loan applicant (existing and past credits, historical defaults, …) as well as information filled in by the applicant (assets, employment data, …). The lender will have additional data coming from their relationship with the applicant or collected through the loan application process.
While this type of data is usually sensitive, loan applicants willingly consent to share their data in order to secure a loan they need. It is up to the regulators then to strike the balance between protecting the privacy of applicants and protecting lenders from insolvency.
Thankfully, the same way technology might create new challenges there are also innovations that can help overcome these. Fully Homomorphic Encryption (FHE) is a technology based in cryptography that allows to perform computations on encrypted data without having to decrypt them. So how can crypto-technology become such a game changer?
Cryptography to the rescue
Applied to credit scoring, FHE can allow any lender to compute a credit score on private applicant’s data without having access to such data.
For a start, regulators are gearing towards more privacy protection for consumers. The current way of operating is not ideal from a privacy point of view but so far has been an acceptable – not satisfying – solution to enable access to credit. Now that technologies such as FHE are more mature, regulators have indicated that they will tighten privacy rules.
As for the consumers’ side, in some cases the applicant doesn’t have any previous credit history. In this scenario, Buy-Now-Pay-Later platforms have to rely on alternative data sources such as bank statements, utility providers and e-commerce purchase history to make a decision. With FHE, all these data can be collected encrypted, enhancing the credit scoring accuracy while protecting the applicant’s privacy
The scoring is not static as lenders continuously monitor their portfolio risks and take corrective measures accordingly. For e.g., a company with a higher risk of bankruptcy creates higher risks for the loans of its employees and suppliers. A lender may therefore detect that it has a high indirect exposure to that company. If it tries to offload some of these loans on the secondary market, it will need to disclose related confidential data to potential buyers that may have their own algorithms and risk exposures. Here again, FHE can help a lender auction the loans it wants to offload without sharing any clear data with potential bidders: bidders will be able to compute their bid based on the loans data without ever seeing the data
The right tools
The efficiency of FHE as a privacy enhancing technology relies in the hands of the developers responsible for creating the infrastructure for organizations and businesses. FHE allows them to do so without seeing the data or having to secure it, as the data would not only be encrypted in transit but also in processing, with the end result also encrypted.
At Zama, we believe in the importance of investing both on the FHE research and on integrating FHE into existing Machine Learning tools, which are widely used to determine credit score. These tools are so simple to use that any data scientist can compile the most relevant credit scoring algorithm even without a previous cryptography knowledge.
Ghazi Ben Amor, VP – Corporate Development at Zama
Ghazi has been working in cyber security for more than 20 years with roles spanning across engineering, strategy, investment and finance. At Zama, he is heading partnerships’ development with a focus on cloud providers, hardware accelerators and financial institutions.
Whether you are a lender, a broker or a regulator, you can contact us to know more about our credit scoring effort on hello@zama.ai